Title:
Crop classification using reduced-dimensionality ndvi time series
Authors:
- Maria Pavlova
- Dmitry Sidorchuk
- Dmitry Bocharov
- Anastasia Sarycheva
Published in:
(2023). ECMS 2023, 37th Proceedings
Edited by: Enrico Vicario, Romeo Bandinelli, Virginia Fani, Michele Mastroianni, European Council for Modelling and Simulation.
DOI: http://doi.org/10.7148/2023
ISSN: 2522-2422 (ONLINE)
ISSN: 2522-2414 (PRINT)
ISSN: 2522-2430 (CD-ROM)
ISBN: 978-3-937436-80-7
ISBN: 978-3-937436-79-1 (CD) Communications of the ECMS Volume 37, Issue 1, June 2023, Florence, Italy June 20th – June 23rd, 2023
DOI:
https://doi.org/10.7148/2023-0306
Citation format:
Maria pavlova, Dmitry sidorchuk, Dmitry bocharov, Anastasia sarycheva (2023). Crop Classification Using Reduced-Dimensionality NDVI Time Series, ECMS 2023, Proceedings Edited by: Enrico Vicario, Romeo Bandinelli, Virginia Fani, Michele Mastroianni, European Council for Modelling and Simulation. doi:10.7148/2023-0306
Abstract:
The paper considers the problem of classification of agricultural crops. As is known, to solve this problem, it is much more efficient to use not instantaneous remote sensing data or calculated vegetation indices, but their historical series. Time series formed by index values for a fixed spatial point at different dates are characterized by a high level of missing values, caused primarily by cloudiness on some dates. A study of known methods of time series approximation has been carried out. The question of whether reducing the dimensionality of the approximated time series can improve the quality of crops classification is also investigated. In the experimental part of the work, NDVI time series calculated from the Sentinel-2 multispectral satellite data were used. The classification of corn, sunflower, wheat and soybeans was studied. The paper shows that UMAP usage for dimensionality reduction leads to 1.5 times increase of classification quality in terms of average the F1-measure compared to using the original dimension data. A new crop classification method based on cubic spline approximation of NDVI time series, extraction of features of low dimension by the UMAP algorithm and their classification by the k nearest neighbors method is proposed.
Full text:
Download full text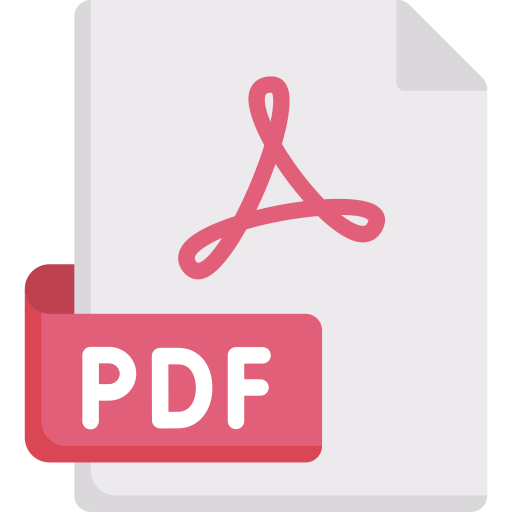