Title:
Hand gesture recognition for human-robot cooperation in manufacturing applications
Authors:
- Stanislaw Hozyn
Published in:
(2023). ECMS 2023, 37th Proceedings
Edited by: Enrico Vicario, Romeo Bandinelli, Virginia Fani, Michele Mastroianni, European Council for Modelling and Simulation.
DOI: http://doi.org/10.7148/2023
ISSN: 2522-2422 (ONLINE)
ISSN: 2522-2414 (PRINT)
ISSN: 2522-2430 (CD-ROM)
ISBN: 978-3-937436-80-7
ISBN: 978-3-937436-79-1 (CD) Communications of the ECMS Volume 37, Issue 1, June 2023, Florence, Italy June 20th – June 23rd, 2023
DOI:
https://doi.org/10.7148/2023-0373
Citation format:
Stanislaw hozyn (2023). Hand Gesture Recognition for Human-Robot Cooperation in Manufacturing Applications, ECMS 2023, Proceedings Edited by: Enrico Vicario, Romeo Bandinelli, Virginia Fani, Michele Mastroianni, European Council for Modelling and Simulation. doi:10.7148/2023-0373
Abstract:
Human-robot cooperation plays an increasingly important role in manufacturing applications. Together, humans and robots display an exceptional skill level that neither can achieve independently. For such cooperation, hand gesture communication using computer vision has been proven to be the most suitable due to the low cost of implementation and flexibility. Therefore, this work focuses on the hand gesture classification problem in view of human and robot collaboration. To facilitate collaboration, six of the most common gestures applicable in manufacturing applications were selected. The first part of the research was devoted to creating an image dataset using the proposed acquisition system. Then, pre-trained neural networks were designed and tested. In this step, the feature extraction approach was adopted, which utilises the representations learned by a previous network to extract meaningful features. The results suggest that all developed pre-trained networks attained high accuracy (above 98,9%). Among them, the VGG19 demonstrated the best performance, achieving accuracy equal to 99,63%. The proposed approach can be easily adapted to recognise a more extensive or different set of gestures. Utilising the proposed vision system and the developed neural network architectures, the adaptation demands only acquiring a set of images and retraining the developed networks.
Full text:
Download full text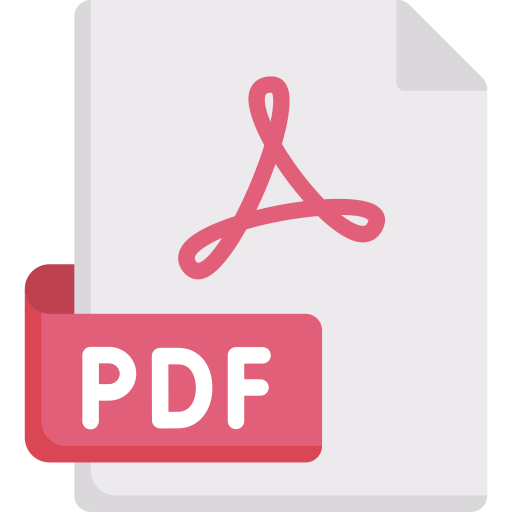