Title:
A light-weight model for run-time battery soc-soh estimation while considering aging
Authors:
- Mohsen Heydarzadeh
- Eero Immonen
- Hashem Haghbayan
- Juha Plosila
Published in:
(2023). ECMS 2023, 37th Proceedings
Edited by: Enrico Vicario, Romeo Bandinelli, Virginia Fani, Michele Mastroianni, European Council for Modelling and Simulation.
DOI: http://doi.org/10.7148/2023
ISSN: 2522-2422 (ONLINE)
ISSN: 2522-2414 (PRINT)
ISSN: 2522-2430 (CD-ROM)
ISBN: 978-3-937436-80-7
ISBN: 978-3-937436-79-1 (CD) Communications of the ECMS Volume 37, Issue 1, June 2023, Florence, Italy June 20th – June 23rd, 2023
DOI:
https://doi.org/10.7148/2023-0466
Citation format:
Mohsen heydarzadeh, Eero immonen, Hashem haghbayan, Juha plosila (2023). A Light-weight Model for Run-time Battery SOC-SOH Estimation While Considering Aging, ECMS 2023, Proceedings Edited by: Enrico Vicario, Romeo Bandinelli, Virginia Fani, Michele Mastroianni, European Council for Modelling and Simulation. doi:10.7148/2023-0466
Abstract:
Batteries are becoming one important part to power varieties of devices including electro-mechanical robots and vehicles. Understanding the behaviour of the battery and its state of charge can help the control systems to significantly improve the decision-making and risk management at run-time, after the device starts its operation. Currently, there is an increased interest in tracking battery dynamics as a function of health in both academia and industry. In this paper, we propose a light-weight approach for modeling the state of charge of lithium-ion (Li-ion) batteries during the life-time of the system. We also consider the battery capacity of charge degradation over its usage. To do that, we use electrical equivalent circuit model (EECM) modeling as the basis for modeling the battery and add the aging model to it to consider the effect of battery usage in the long term. Experimental results show that our proposed technique successfully estimates the battery state of charge at different states of health for the National Aeronautics and Space Administration (NASA) randomized usage battery dataset in comparison with the state-of-the-art. The obtained estimation error in the worst case is 2.2%.
Full text:
Download full text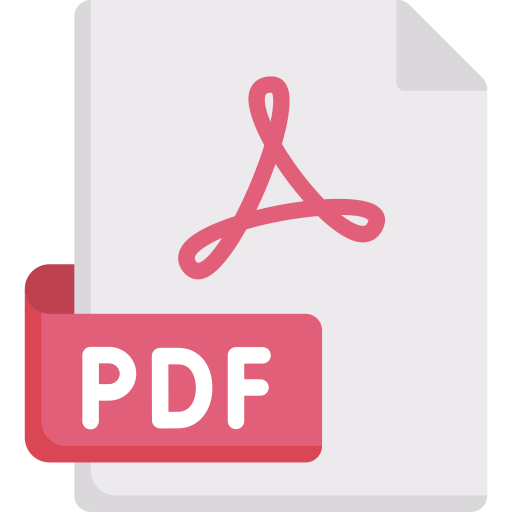